Wikipedia:Wikipedia Signpost/2018-07-31/Recent research
Different Wikipedias use different images; editing contests more successful than edit-a-thons
A monthly overview of recent academic research about Wikipedia and other Wikimedia projects, also published as the Wikimedia Research Newsletter.
Diverse image usage across languages
- Reviewed by Morten Warncke-Wang
A paper[1] presented at the recent ICWSM conference studies image usage across 25 of the larger Wikipedia language editions. Whereas diversity of the text of Wikipedia's articles across language editions has received much attention from researchers, studies of image and other media usage is rare. The paper has two main research questions:
- What is the diversity of visual encyclopedic knowledge across language editions of Wikipedia?
- How does the diversity in visual knowledge compare to the diversity of textual encyclopedia knowledge?
The paper chose to study 25 specific language editions to enable direct comparisons to prior work on textual diversity. Their methodology only examines image usage in articles that are not redirects, not disambiguation pages, and were not created by a bot (thereby excluding, for example, the Swedish Wikipedia's extensive amount of bot-created pages), resulting in a dataset of more than 23 million articles. Furthermore, they develop a method to filter out images that are frequently included based on templates, such as navigation boxes or stub templates, as these often do not reflect visual encyclopedic knowledge. Previous studies of textual diversity similarly removed templated-based links.
To enable exploration of the image use across languages, the authors developed the WikiImg Drive tool, a tool that visualizes image usage for any concept found in multiple Wikipedia editions. The tool provides information on how many editions has an article about a given concept, how many images are found in those articles, and shows a chord diagram to visualize image usage across the languages (an example diagram is shown below). Users can then get further information about what the used images are, and which specific editions use a given image.

The paper studied diversity of visual encyclopedic knowledge in two ways: image diversity across language editions, and image diversity within coverage of a concept. When it comes to image diversity across language editions, they find that more than 67.4% of all images only appear in a single language edition. The proportion of images used in multiple editions is relatively small and decreasing quickly: only 14.1% of images appear in two editions, and in all only 142 images (0.0014%) were used in all 25 language editions. The majority of these "global images" are either portraits or other images showing a specific person.
For image diversity within concepts, they made pairwise comparisons and calculated averages, finding a range from 22.2% (German and Indonesian) to 75.6% (Hungarian and Romanian). This means that, on average, more than 24% of images used in an article in a specific language will be unique relative to an article about the same concept in a different language, and that for some languages this rises to almost 80%.
Lastly, when comparing visual diversity to textual diversity, they find that the overall degree of diversity is roughly similar. At the far ends of the diversity scale, there are some clear differences: 67.4% of images are only used in a single language edition, whereas 73.5% of concepts have an article in only one language edition. This reverses on the opposite end of the scale: 0.13% of concepts are "global concepts" (found in all 25 editions), whereas only 0.0014% of the images are "global images".
The paper discusses what may drive the larger diversity in image usage. Cultural diversity can lead to significant differences in how one might want to illustrate a concept. There are also examples of language editions using variations of the same image, meaning that further image analysis would be necessary in order to identify those. Lastly, they discuss whether images having English names on Commons might enable cross-language usage. Previous research from 2012 (where this reviewer was one of the authors) found that English is the primary language from which translations were made,[supp 1] and we have also covered research in May 2016 that found English to still be the lingua franca of Wikipedia.
Furthermore, the paper discusses how the diversity in image usage can affect algorithms and AI trained on Wikipedia data, cautioning that using images from a single edition will likely result in a biased view. The paper points out that gathering images from all language editions is relatively straightforward and should therefore be the preferred approach.
Filling knowledge gaps: PDFs as "boundary objects" between experts and Wikipedia
- Reviewed by Morten Warncke-Wang
What strategies have success when seeking to fill knowledge gaps in peer-produced content? In a recent paper titled "Beyond notification: Filling gaps in peer production projects",[2] Ford et al. studied several approaches aiming to improve coverage in articles relevant to teachers in South African primary schools. A committee of scholars and researchers in ICT and education—especially primary school education—along with teachers, parents, and Wikipedia experts, identified 183 articles in the English Wikipedia that are relevant to the South African national primary school curriculum. Five strategies for soliciting improvements to these 183 articles were then tested and evaluated with regard to whether articles were improved, and if the strategy was helpful in bringing new contributors to Wikipedia:
- Edit competitions were relatively successful. Articles with few online sources or requiring specialized knowledge were unlikely to be improved, and the competitions did not result in newcomers editing.
- Edit-a-thons were not successful as no articles were improved during those.
- Notifications proved largely unsuccessful. These notifications were sent to WikiProjects, and regardless of whether there appeared to be activity within the project or articles related to it, the notifications tended to be ignored.
- Reaching out to academics to write topic pages that can later be moved on to Wikipedia as articles was unsuccessful. One academic responded negatively, noting that they did not recognize Wikipedia as a legitimate academic enterprise.
- Eliciting and responding to expert reviews resulted in a low overall number of improved articles, but had the most sustained engagement and the highest quality results. The experts would get a PDF of the Wikipedia article and review it. The team would then copy the comments onto the article's talk page, and use OTRS to verify that the comments were appropriately licensed by the expert. Wikipedia contributors could then respond by incorporating changes to the article or discuss the review on the talk page.
In the paper, Ford et al. discuss how the PDFs can be seen as a form of "boundary objects" that allow for a negotiation between the workflow and epistemological paradigms of the experts and Wikipedia, and that this negotiation is necessary in order to facilitate collaboration. They also argue that expanding the collaboration between experts and Wikipedia contributors is an important strategy to close the knowledge gaps in the encyclopedia.
Contributor experience and article quality
- Reviewed by Morten Warncke-Wang
A short paper recently published in the Journal of Medical Internet Research studies the "Effects of Contributor Experience on the Quality of Health-Related Wikipedia Articles".[3] Using a dataset of 18,805 articles from the Health and Fitness portal on the English Wikipedia, the paper compares those articles that were at some point tagged with a template indicating a quality flaw (also called "cleanup templates") to those that never contained such a template. The goal is to understand to what extent contributor experience, in the form of average number of edits made or number of articles edited by contributors to these articles, correlates with the presence of these cleanup templates. Only the number of articles edited was found to have a significant relationship, and contributors to non-tagged articles had a higher average number of articles edited. The authors discuss these findings in relation to ensuring that articles about medical topics on Wikipedia are of high quality.
The paper's limitation section discusses the operationalization of article quality used, citing early work on predicting article quality and suggesting that the methodology could be improved by incorporating multiple quality factors. This resonated with this reviewer, who has both done extensive research in this area and reviewed other work for this newsletter. There are two papers that appear particularly relevant in this case: we covered one using a deep learning approach last year, and a second paper used the ORES API to measure the development of article quality across time enabling a demonstration of the Keilana Effect.
Teahouse
- Reviewed by Kudpung
- "Evaluating the Impact of the Wikipedia Teahouse on Newcomer Retention"[4]
- Aaron Halfaker is a WMF employee and edits Wikipedia as EpochFail. Jonathan Morgan is a WMF employee and, while editing as Jtmorgan, is a Teahouse Host.
From the abstract: "[F]ew interventions employed to increase newcomer retention over the long term by improving aspects of the onboarding experience have demonstrated success. This study presents an evaluation of the impact of one such intervention, the Wikipedia Teahouse, on new editor survival. In a controlled experiment, we find that new editors invited to the Teahouse are retained at a higher rate than editors who do not receive an invite. The effect is observed for both low- and high-activity newcomers, and for both short- and long-term survival."
Can there ever be a solution to the dwindling number of new and active users? In their paper, Halfaker and Morgan explain that "[s]o far, neither purely social or purely technical efforts have been shown to be effective at providing effective socialization at a scale that leads to a substantial increase in the number of new editors who go on to become Wikipedians." They examine the Teahouse which they describe as "one of the most potentially impactful retention mechanisms that has been attempted". Their research demonstrates that "new editors who are invited to the Teahouse are significantly more likely to continue contributing after three weeks, two months, and six months than a similar cohort who were not invited."
Comparing it with the Wikipedia Adventure new editor training system developed in 2013, which—although often used—did not show any long-term impact, the Teahouse (created in 2012) "combined social and technical components to provide comprehensive socialization on a large scale, and was designed to promote long-term retention." Their study evaluates "the effect of invitation to the Teahouse, rather than participation in the Teahouse." Both the 24-hour and 5-edit threshold before issuing a Teahouse invitation, while avoiding vandalism-only accounts, may result in "many good-faith newcomers being denied the opportunity for positive socialization", they say.
"ORES", they explain, "provides powerful predictive models that can accurately discriminate between damaging and a non-damaging edits, and between malicious edits and edits that were made in good faith, even if they introduce errors or fail to comply with policies." The authors consider that "most new editors" receive "overwhelmingly negative and alienating experience [...] when they first join Wikipedia," but they do not appear to draw on any data for this assumption.
Briefly
Research presentations at Wikimania 2018
- Summarized by Morten Warncke-Wang and Tilman Bayer
One of the presentations at the recent Wikimania 2018 conference was on the "State of Wikimedia Research 2017–2018". An almost yearly occurrence since 2009, this presentation gives a quick look into the overarching themes in research published about Wikimedia projects over the previous year. This year's presentation (slides) is now available on YouTube, and covers five main themes: images & media, talk pages, multilingual comparisons, non-participation (who is not contributing?), and Wikipedia as a source of data. The first of these highlights the "Tower_of_Babel.jpg" paper also covered above.
A keynote titled "Creating Knowledge Equity and Spatial Justice on Wikipedia" (summary, slides) by Martin Dittus, a data scientist at the Oxford Internet Institute, featured various results about the geographical distribution of geotagged Wikipedia articles and IP edits, partly from earlier research by Dittus' colleague Mark Graham and others (cf. earlier coverage). Other presentations included:
- "The State of Research in Knowledge Gaps" (video), showcasing various research results and technology projects by the Wikimedia Foundation's research team and its academic collaborators;
- "Research on gender gap in Wikipedia: What do we know so far?" (video, slides); and
- A lightning talk (video, slides, poster) about the "Wikipedia Cultural Diversity Observatory" (WCDO) project, which likewise uses geolocation coordinates to associate articles with cultural contexts, but also draws on other data such as article categories and Wikidata properties to overcome some of the shortcomings of the geotagging data.
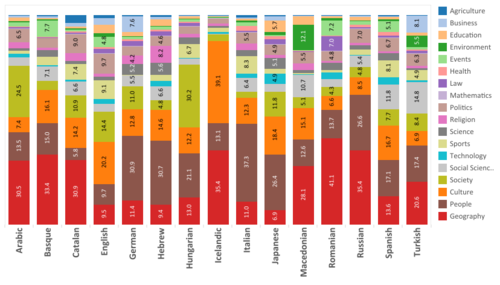
Other recent publications
Other recent publications that could not be covered in time for this issue include the items listed below. Contributions are always welcome for reviewing or summarizing newly published research.
- Compiled by Kudpung and Tilman Bayer
Vandalism
- "Vandalism on Collaborative Web Communities: An Exploration of Editorial Behaviour in Wikipedia"[5]
The study found that "most vandalisms [on Wikipedia] were reverted within five minutes" on average and that "the majority of the articles targeted [with vandalism] are related to Politics (29.4%), followed by Culture (26.4%), Music (23.5%), Animals (11.7%) and History (8.8%)."
Simple English
- "Evaluating lexical coverage in the Simple English Wikipedia articles: a corpus-driven study"[6]
From the paper: "[Simple English Wikipedia] articles require surprisingly large vocabularies to comprehend, comparable to that required to read standard Wikipedia articles."
"Open algorithmic systems: lessons on opening the black box from Wikipedia"
From the abstract:[7] "This paper reports from a multi-year ethnographic study of automated software agents in Wikipedia, where bots play key roles in moderation and gatekeeping. Automated software agents are playing increasingly important roles in how networked publics are governed and gatekept, with internet researchers increasingly focusing on the politics of algorithms. [...] In most platforms, algorithmic systems are developed in-house, where there are few measures for public accountability or auditing, much less the ability for publics to shape the design or operation of such systems. However, Wikipedia's model presents a compelling alternative, where members of the editing community heavily participate in the design and development of such algorithmic systems."
"High-quality standards in information presentation are not globally shared" across Wikipedia languages
- "Cultural diversity of quality of information on Wikipedias"[8]
From the abstract: "This article explores the relationship between linguistic culture and the preferred standards of presenting information based on article representation in major Wikipedias. Using primary research analysis of the number of images, references, internal links, external links, words, and characters, as well as their proportions in Good and Featured articles on the eight largest Wikipedias, we discover a high diversity of approaches and format preferences, correlating with culture. We demonstrate that high-quality standards in information presentation are not globally shared and that in many aspects, the language culture's influence determines what is perceived to be proper, desirable, and exemplary for encyclopedic entries."
Revert behavior differs between "political" and "unpolitical" articles
- "Case study in political user behavior on Wikipedia"[9]
From the paper:
“ | We [selected] six broad categories of Wikipedia articles likely to include more political bias. These include political activism (Damascus Declaration, Gaza flotilla raid), political controversies (Christmas controversy, Tibetan sovereignty debate), political riots (Anti-austerity movement in Greece, 27th G8 summit), political party founders (Abraham Lincoln, Julian Assange), political theories (Agrarianism, Liberal conservatism) and political schisms (Australian Labour split, Sino–Soviet split). The reason for picking six categories was that the categories chosen should be strongly political and that the sample should not be too small.
[... Both] political and unpolitical articles [...] seem to follow a general pyramid structure, with a majority of the users contributing an occasional revision and a few contributing many revisions. The categories differ however in regard to reverts, a sub group of revisions. The political articles across all categories had a more significant amount of medium frequent contributors joining the process, leading to a more even distribution. This is in contrast to the unpolitical articles where nearly half the reverts were done by the most frequent contributors (the Experts). |
” |
How German Wikipedians coin words that describe unwanted editing behaviors as diseases
- "Combinatorics of the suffix -itis on talk pages of Wikipedia: A word formation pattern for the discursive regulation in the collaborative knowledge production" (in German)[10]
From the English abstract: "The study reveals that -itis is a highly productive suffix in meta(-linguistic) discourses of the online-encyclopaedia: Wikipedia authors using word formation products with the suffix -itis (e.g. Newstickeritis or WhatsAppitis) try to standardise the collaborative knowledge production with the help of these linguistic innovations. The corpus analysis delivers evidence for the fact that certain linguistic innovations and special types of word formation characterise the community of Wikipedia authors and their discourse traditions."
"Linking ImageNet WordNet Synsets with Wikidata"
From the abstract:[11] "The linkage of ImageNet WordNet synsets to Wikidata items will leverage deep learning algorithm with access to a rich multilingual knowledge graph. [...] I show an example on how the linkage can be used in a deep learning setting with real-time image classification and labeling in a non-English language and discuss what opportunities lies ahead."
"Capturing the influence of geopolitical ties from Wikipedia with reduced Google matrix"
From the abstract:[12] "[We] show that meaningful results on the influence of country ties can be extracted from the hyperlinked structure of Wikipedia. We leverage a novel stochastic matrix representation of Markov chains of complex directed networks called the reduced Google matrix theory. [...] We apply this analysis to two chosen sets of countries (i.e. the set of 27 European Union countries and a set of 40 top worldwide countries). We [...] can exhibit easily very meaningful information on geopolitics from five different Wikipedia editions (English, Arabic, Russian, French and German)." (See also earlier by some of the same authors: "Multi-cultural Wikipedia mining of geopolitics interactions leveraging reduced Google matrix analysis".)
AI assessment of article quality using deep learning
- "A Hybrid Model for Quality Assessment of Wikipedia Articles"[13]
From the abstract: "We explore the task [of document quality assessment] in the context of a Wikipedia article assessment task, and propose a hybrid approach combining deep learning with features proposed in the literature. Our method achieves 6.5% higher accuracy than the state of the art in predicting the quality classes of English Wikipedia articles over a novel dataset of around 60k Wikipedia articles." (See also earlier coverage in August 2017 of related research by a different team: "Improved article quality predictions with deep learning".)
"Social capital" of editors has a "significant impact" on article quality
- "Using big data and network analysis to understand Wikipedia article quality"[14]
From the abstract: "The research reported in this paper focuses on the question of why Wikipedia articles are different in quality. [...] We focus on three major types of social capital with respect to teams of contributors working on Wikipedia articles: internal bonding, external bridging and functional diversity. Through a social network analysis of these articles based on a dataset extracted from its edit history, our research finds that all three types of social capital have a significant impact on their quality. In addition, we found that internal bonding interacts positively with external bridging resulting in a multiplier effect on article quality."
References
- ^ He, Shiqing; Lin, Allen Yilun; Adar, Eytan; Hecht, Brent (15 June 2018). "The_Tower_of_Babel.jpg: Diversity of Visual Encyclopedic Knowledge Across Wikipedia Language Editions" (PDF). Proceedings of the International AAAI Conference on Web and Social Media. The 12th International AAAI Conference on Web and Social Media (ICWSM-18). Vol. 12. Palo Alto, California: Association for the Advancement of Artificial Intelligence. pp. 102–111. eISSN 2334-0770. ISBN 978-1-57735-798-8. ISSN 2162-3449. Archived from the original on 30 July 2018. Retrieved 30 July 2018.
{{cite conference}}
: External link in
(help)|conference=
- ^ Ford, Heather; Pensa, Iolanda; Devouard, Florence; Pucciarelli, Marta; Botturi, Luca (24 March 2018). "Beyond notification: Filling gaps in peer production projects". New Media & Society. 20 (3). SAGE Publications: 1–19. doi:10.1177/1461444818760870. eISSN 1461-7315. ISSN 1461-4448. OCLC 7782926975.
Ford, Heather; Pensa, Iolanda; Devouard, Florence; Pucciarelli, Marta; Botturi, Luca (27 March 2018). "Beyond notification: Filling gaps in peer production projects". SocArxiv (Preprint). Center for Open Science. doi:10.17605/OSF.IO/QN5XD.
- ^ Holtz, Peter; Fetahu, Besnik; Kimmerle, Joachim (10 May 2018). "Effects of Contributor Experience on the Quality of Health-Related Wikipedia Articles". Journal of Medical Internet Research. 20 (5). JMIR Publications. e171. doi:10.2196/jmir.9683. eISSN 1438-8871. ISSN 1439-4456. OCLC 7587362244. PMC 5968213. PMID 29748161. Archived from the original on 30 July 2018. Retrieved 30 July 2018.
- ^ Morgan, Jonathan T.; Halfaker, Aaron (22 August 2018). "Evaluating the impact of the Wikipedia Teahouse on newcomer socialization and retention" (PDF). Proceedings of the International Symposium on Open Collaboration. The 14th International Symposium on Open Collaboration (OpenSym '18). Vol. 14. New York: Association for Computing Machinery. Article 20. doi:10.1145/3233391.3233544. ISBN 978-1-4503-5936-8. Archived (PDF) from the original on 30 July 2018. Retrieved 30 July 2018.
{{cite conference}}
: External link in
(help)|conference=
Morgan, Jonathan T.; Halfaker, Aaron (19 April 2018). "Evaluating the impact of the Wikipedia Teahouse on newcomer socialization and retention". SocArxiv (Preprint). Center for Open Science. doi:10.17605/OSF.IO/8QSV6.
- ^ Alkharashi, Abdulwhab; Jose, Joeman (26 June 2018). "Vandalism on Collaborative Web Communities: An Exploration of Editorial Behaviour in Wikipedia" (PDF). In Tramullas, Jesús; Trillo-Lado, Raquel; Nogueras-Iso, Javier (eds.). Proceedings of the Spanish Conference on Information Retrieval. V Congreso Español de Recuperación de Información [The 5th Spanish Conference on Information Retrieval] (CERI '18). International Conference Proceedings Series. Vol. 5. New York: Association for Computing Machinery. Article 8. doi:10.1145/3230599.3230608. ISBN 978-1-4503-6543-7. Archived (PDF) from the original on 30 July 2018. Retrieved 30 July 2018 – via University of Glasgow.
{{cite conference}}
: External link in
(help)|conference=
- ^ Hendry, Clinton; Sheepy, Emily (3 December 2017). "Evaluating lexical coverage in Simple English Wikipedia articles: a corpus-driven study." (PDF). In Borthwick, Kate; Bradley, Linda; Thouësny, Sylvie (eds.). CALL in a climate of change: adapting to turbulent global conditions – short papers from EUROCALL 2017 (PDF) (E-book) (1st ed.). Dublin, Ireland; Voillans, France: Research Publishing. pp. 146–150. doi:10.14705/rpnet.2017.eurocall2017.9782490057047. ISBN 978-2-490057-04-7. OCLC 7585336027. Archived (PDF) from the original on 30 July 2018. Retrieved 30 July 2018.
- ^ Geiger, R. Stuart; Halfaker, Aaron (5–8 October 2016). "Open algorithmic systems: lessons on opening the black box from Wikipedia". Selected Papers of Internet Research 2016. The 17th Annual Conference of the Association of Internet Researchers (#AoIR2016). Vol. 6. Berlin, Germany: Association of Internet Researchers (published 16 May 2017). ISSN 2162-3317. Retrieved 30 July 2018.
{{cite conference}}
: External link in
(help)|conference=
- ^ Jemielniak, Dariusz; Wilamowski, Maciej (7 August 2017). "Cultural Diversity of Quality of Information on Wikipedias" (PDF). Journal of the Association for Information Science and Technology. 68 (10). Wiley-Blackwell: 2460–2470. doi:10.1002/asi.23901. eISSN 2330-1643. ISSN 2330-1635. LCCN 2013203451. OCLC 7150253054. Archived (PDF) from the original on 31 July 2018. Retrieved 30 July 2018.
- ^ Neppare, Christoffer; Blomberg, Pontus (11 May 2016). Fallstudie i politiskt användarbeteende på Wikipedia [Case study in political user behavior on Wikipedia] (PDF). School of Computer Science and Communication (Bachelor thesis). Stockholm, Sweden: KTH Royal Institute of Technology (published 24 May 2016). Archived from the original (PDF) on 31 July 2018. Retrieved 31 July 2018.
- ^ Gredel, Eva (12 April 2018). "Itis-Kombinatorik auf den Diskussionsseiten der Wikipedia: Ein Wortbildungsmuster zur diskursiven Normierung in der kollaborativen Wissenskonstruktion" [Combinatorics of the suffix -itis on talk pages of Wikipedia: A word formation pattern for the discursive regulation in the collaborative knowledge production]. Zeitschrift für Angewandte Linguistik [Journal for Applied Linguistics] (in German). 68 (1). Walter de Gruyter: 35–72. doi:10.1515/zfal-2018-0003. eISSN 2190-0191. ISSN 1433-9889. OCLC 7468452272.
- ^ Nielsen, Finn Årup (23 April 2018). "Linking ImageNet WordNet Synsets with Wikidata" (PDF). In Champin, Pierre-Antoine; Gandon, Fabien; Médini, Lionel; Lalmas, Mounia; Ipeirotis, Panagiotis G. (eds.). Companion Proceedings of the The Web Conference 2018. The Web Conference 2018 (WWW '18). Geneva, Switzerland: International World Wide Web Conference Committee. pp. 1809–1814. arXiv:1803.04349v1. doi:10.1145/3184558.3191645. ISBN 978-1-4503-5640-4. Archived (PDF) from the original on 31 July 2018. Retrieved 31 July 2018.
{{cite conference}}
: External link in
(help)|conference=
- ^ Zant, Samer El; Jaffrès-Runser, Katia; Shepelyansky, Dima (13 March 2018). "Capturing the influence of geopolitical ties from Wikipedia with reduced Google matrix". arXiv:1803.05336v1 [cs.SI].
- ^ Shen, Aili; Qi, Jianzhong; Baldwin, Timothy (December 2017). "A Hybrid Model for Quality Assessment of Wikipedia Articles" (PDF). In Wong, Jojo Sze-Meng; Haffari, Gholamreza (eds.). Proceedings of the Australasian Language Technology Association Workshop. 15th Annual Workshop of the Australasian Language Technology Association (ALTA '17). Vol. 15. Australasian Language Technology Association. pp. 43–52. ISSN 1834-7037. Archived from the original on 31 July 2018. Retrieved 31 July 2018.
{{cite conference}}
: External link in
(help)|conference=
- ^ Liu, Jun; Ram, Sudha (16 February 2018). "Using big data and network analysis to understand Wikipedia article quality". Data & Knowledge Engineering. 115. Elsevier (published May 2018): 80–93. doi:10.1016/j.datak.2018.02.004. eISSN 1872-6933. ISSN 0169-023X. LCCN 90649274. OCLC 7321135118.
Supplementary references
- ^ Warncke-Wang, Morten; Uduwage, Anuradha; Dong, Zhenhua; Riedl, John (27 August 2012). "In Search of the Ur-Wikipedia: Universality, Similarity, and Translation in the Wikipedia Inter-language Link Network" (PDF). In Lampe, Cliff; Cosley, Dan (eds.). Proceedings of the International Symposium on Wikis and Open Collaboration. 8th International Symposium on Wikis and Open Collaboration (WikiSym '12). Vol. 8. New York: Association for Computing Machinery. Article 10. doi:10.1145/2462932.2462959. ISBN 978-1-4503-1605-7. OCLC 5132192281. Archived (PDF) from the original on 30 July 2018. Retrieved 30 July 2018.
{{cite conference}}
: External link in
(help)|conference=
Discuss this story