Human agency and infection rates: Implications for social distancing during epidemics
- PMID: 33320880
- PMCID: PMC7737959
- DOI: 10.1371/journal.pone.0243699
Human agency and infection rates: Implications for social distancing during epidemics
Abstract
Social distancing is an important measure in controlling epidemics. This paper presents a simple theoretical model focussed on the implications of the wide range in interaction rates between individuals, both within the workplace and in social settings. The model is based on well-mixed populations and so is not intended for studying geographic spread. The model shows that epidemic growth rate is largely determined by the upper interactivity quantiles of society, implying that the most efficient methods of epidemic control are interaction capping approaches rather than overall reductions in interaction. The theoretical model can also be applied to look at aspects of the dynamics of epidemic progression under various scenarios. The theoretical model suggests that with no intervention herd immunity would be achieved with a lower overall infection rate than if variation in interaction rate is ignored, because by this stage almost all the most interactive members of society would have had the infection; however the overall mortality with such an approach is very high. Scenarios for mitigation and suppression suggest that, by using interactivity capping, it should be possible to control an epidemic without extreme sanctions on the majority of the population if R0 of the uncontrolled infection is 2.4. However to control the infection rate to a specific level will always require measures to be switched on and off and for this reason elimination is likely to be a less costly policy in the long run. While social distancing alone can be used for elimination, it would not on its own be an efficient mechanism to prevent reinfection. The use of robust testing, quarantining, and contact tracing would strengthen any social distancing measures, speed up elimination, and be a better tool for the prevention of infection or reinfection. Because the analysis presented here is theoretical, and not data-driven, it is intended to be a stimulus for further data-collection, particularly on individual interactivity levels, and for more comprehensive modelling which takes account of the type of heterogeneity discussed here. While there are some clear lessons from the simple model presented here, policy makers should have these tested and validated by epidemiological specialists before acting on them.
Conflict of interest statement
The author has declared that no competing interests exist.
Figures

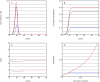
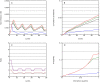
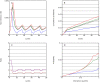


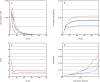
Similar articles
-
Spontaneous social distancing in response to a simulated epidemic: a virtual experiment.BMC Public Health. 2015 Sep 28;15:973. doi: 10.1186/s12889-015-2336-7. BMC Public Health. 2015. PMID: 26415861 Free PMC article.
-
Chopping the tail: How preventing superspreading can help to maintain COVID-19 control.Epidemics. 2021 Mar;34:100430. doi: 10.1016/j.epidem.2020.100430. Epub 2020 Dec 21. Epidemics. 2021. PMID: 33360871 Free PMC article.
-
Discontinuous epidemic transition due to limited testing.Nat Commun. 2021 May 10;12(1):2586. doi: 10.1038/s41467-021-22725-9. Nat Commun. 2021. PMID: 33972522 Free PMC article.
-
Application of optimal control to the onchocerciasis transmission model with treatment.Math Biosci. 2018 Mar;297:43-57. doi: 10.1016/j.mbs.2017.11.009. Epub 2017 Nov 23. Math Biosci. 2018. PMID: 29175094 Review.
-
Tuberculosis.In: Holmes KK, Bertozzi S, Bloom BR, Jha P, editors. Major Infectious Diseases. 3rd edition. Washington (DC): The International Bank for Reconstruction and Development / The World Bank; 2017 Nov 3. Chapter 11. In: Holmes KK, Bertozzi S, Bloom BR, Jha P, editors. Major Infectious Diseases. 3rd edition. Washington (DC): The International Bank for Reconstruction and Development / The World Bank; 2017 Nov 3. Chapter 11. PMID: 30212088 Free Books & Documents. Review.
References
-
- Ferguson N, Laydon D, Nedjati Gilani G, Imai N, Ainslie K, Baguelin M, et al. Report 9: Impact of non-pharmaceutical interventions (NPIs) to reduce COVID19 mortality and healthcare demand. Imperial College; London; 2020. Available from: http://hdl.handle.net/10044/1/77482.
-
- Pastor-Satorras R, Castellano C, Van Mieghem P, Vespignani A. Epidemic processes in complex networks. Rev Mod Phys. 2015;87:925–979. 10.1103/RevModPhys.87.925 - DOI
-
- Kissler SM, Klepac P, Tang M, Conlan AJK, Gog JR. Sparking “The BBC Four Pandemic”: Leveraging citizen science and mobile phones to model the spread of disease. bioRxiv. 2018. 10.1101/479154 - DOI
MeSH terms
Grants and funding
LinkOut - more resources
Full Text Sources