A multi-modal single-cell and spatial expression map of metastatic breast cancer biopsies across clinicopathological features
- PMID: 39478111
- PMCID: PMC11564109
- DOI: 10.1038/s41591-024-03215-z
A multi-modal single-cell and spatial expression map of metastatic breast cancer biopsies across clinicopathological features
Abstract
Although metastatic disease is the leading cause of cancer-related deaths, its tumor microenvironment remains poorly characterized due to technical and biospecimen limitations. In this study, we assembled a multi-modal spatial and cellular map of 67 tumor biopsies from 60 patients with metastatic breast cancer across diverse clinicopathological features and nine anatomic sites with detailed clinical annotations. We combined single-cell or single-nucleus RNA sequencing for all biopsies with a panel of four spatial expression assays (Slide-seq, MERFISH, ExSeq and CODEX) and H&E staining of consecutive serial sections from up to 15 of these biopsies. We leveraged the coupled measurements to provide reference points for the utility and integration of different experimental techniques and used them to assess variability in cell type composition and expression as well as emerging spatial expression characteristics across clinicopathological and methodological diversity. Finally, we assessed spatial expression and co-localization features of macrophage populations, characterized three distinct spatial phenotypes of epithelial-to-mesenchymal transition and identified expression programs associated with local T cell infiltration versus exclusion, showcasing the potential of clinically relevant discovery in such maps.
© 2024. The Author(s).
Conflict of interest statement
Figures
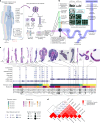
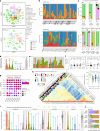
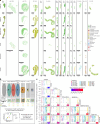
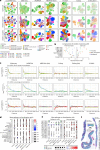
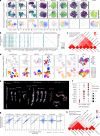
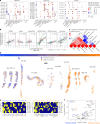
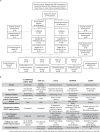
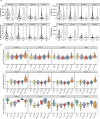
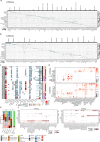
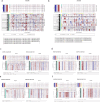
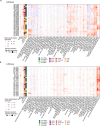
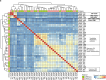
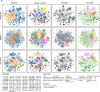
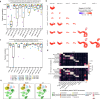
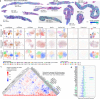
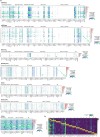
Similar articles
-
Core needle biopsy of breast cancer tumors increases distant metastases in a mouse model.Neoplasia. 2014 Nov 20;16(11):950-60. doi: 10.1016/j.neo.2014.09.004. eCollection 2014 Nov. Neoplasia. 2014. PMID: 25425969 Free PMC article.
-
Study on the mechanism of heterogeneous tumor-associated macrophages in three subtypes of breast cancer through the integration of single-cell RNA sequencing and in vitro experiments.Mol Biol Rep. 2024 Jun 1;51(1):720. doi: 10.1007/s11033-024-09665-5. Mol Biol Rep. 2024. PMID: 38824268
-
Intratumoral heterogeneity of macrophages and fibroblasts in breast cancer is associated with the morphological diversity of tumor cells and contributes to lymph node metastasis.Immunobiology. 2017 Apr;222(4):631-640. doi: 10.1016/j.imbio.2016.11.012. Epub 2016 Nov 27. Immunobiology. 2017. PMID: 27916281
-
The role of tumor-associated macrophages in breast cancer progression (review).Int J Oncol. 2013 Jul;43(1):5-12. doi: 10.3892/ijo.2013.1938. Epub 2013 May 14. Int J Oncol. 2013. PMID: 23673510 Free PMC article. Review.
-
Single-Cell RNA-Sequencing: Opening New Horizons for Breast Cancer Research.Int J Mol Sci. 2024 Aug 31;25(17):9482. doi: 10.3390/ijms25179482. Int J Mol Sci. 2024. PMID: 39273429 Free PMC article. Review.
References
MeSH terms
Grants and funding
- 1P50CA168504/U.S. Department of Health & Human Services | National Institutes of Health (NIH)
- 2019_A70/Else Kröner-Fresenius-Stiftung (Else Kroner-Fresenius Foundation)
- P50 CA168504/CA/NCI NIH HHS/United States
- HHSN261201500003C/CA/NCI NIH HHS/United States
- 17095/Conquer Cancer Foundation (Conquer Cancer Foundation of the American Society of Clinical Oncology)
LinkOut - more resources
Full Text Sources
Medical