A single-cell landscape of high-grade serous ovarian cancer
- PMID: 32572264
- PMCID: PMC7723336
- DOI: 10.1038/s41591-020-0926-0
A single-cell landscape of high-grade serous ovarian cancer
Abstract
Malignant abdominal fluid (ascites) frequently develops in women with advanced high-grade serous ovarian cancer (HGSOC) and is associated with drug resistance and a poor prognosis1. To comprehensively characterize the HGSOC ascites ecosystem, we used single-cell RNA sequencing to profile ~11,000 cells from 22 ascites specimens from 11 patients with HGSOC. We found significant inter-patient variability in the composition and functional programs of ascites cells, including immunomodulatory fibroblast sub-populations and dichotomous macrophage populations. We found that the previously described immunoreactive and mesenchymal subtypes of HGSOC, which have prognostic implications, reflect the abundance of immune infiltrates and fibroblasts rather than distinct subsets of malignant cells2. Malignant cell variability was partly explained by heterogeneous copy number alteration patterns or expression of a stemness program. Malignant cells shared expression of inflammatory programs that were largely recapitulated in single-cell RNA sequencing of ~35,000 cells from additionally collected samples, including three ascites, two primary HGSOC tumors and three patient ascites-derived xenograft models. Inhibition of the JAK/STAT pathway, which was expressed in both malignant cells and cancer-associated fibroblasts, had potent anti-tumor activity in primary short-term cultures and patient-derived xenograft models. Our work contributes to resolving the HSGOC landscape3-5 and provides a resource for the development of novel therapeutic approaches.
Figures
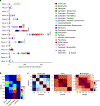
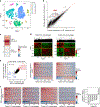
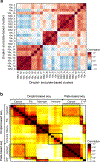
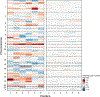
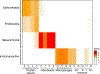
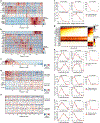
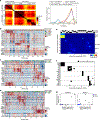
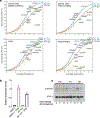
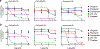
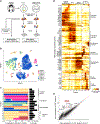
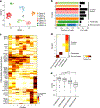
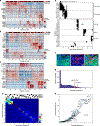
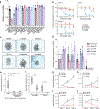
Similar articles
-
Molecular profiling and clinical outcome of high-grade serous ovarian cancer presenting with low- versus high-volume ascites.Biomed Res Int. 2014;2014:367103. doi: 10.1155/2014/367103. Epub 2014 May 25. Biomed Res Int. 2014. PMID: 24982872 Free PMC article.
-
Malignant ascites occurs most often in patients with high-grade serous papillary ovarian cancer at initial diagnosis: a retrospective analysis of 191 women treated at Bayreuth Hospital, 2006-2015.Arch Gynecol Obstet. 2019 Feb;299(2):515-523. doi: 10.1007/s00404-018-4952-9. Epub 2018 Nov 10. Arch Gynecol Obstet. 2019. PMID: 30415435
-
Development and Validation of the Gene Expression Predictor of High-grade Serous Ovarian Carcinoma Molecular SubTYPE (PrOTYPE).Clin Cancer Res. 2020 Oct 15;26(20):5411-5423. doi: 10.1158/1078-0432.CCR-20-0103. Epub 2020 Jun 17. Clin Cancer Res. 2020. PMID: 32554541 Free PMC article.
-
Studying platinum sensitivity and resistance in high-grade serous ovarian cancer: Different models for different questions.Drug Resist Updat. 2016 Jan;24:55-69. doi: 10.1016/j.drup.2015.11.005. Epub 2015 Nov 26. Drug Resist Updat. 2016. PMID: 26830315 Review.
-
The high-grade serous ovarian cancer metastasis and chemoresistance in 3D models.Biochim Biophys Acta Rev Cancer. 2024 Jan;1879(1):189052. doi: 10.1016/j.bbcan.2023.189052. Epub 2023 Dec 12. Biochim Biophys Acta Rev Cancer. 2024. PMID: 38097143 Review.
Cited by
-
ClassifieR 2.0: expanding interactive gene expression-based stratification to prostate and high-grade serous ovarian cancer.BMC Bioinformatics. 2024 Nov 21;25(1):362. doi: 10.1186/s12859-024-05981-6. BMC Bioinformatics. 2024. PMID: 39574035 Free PMC article.
-
Reversible downregulation of MYC in a spheroid model of metastatic epithelial ovarian cancer.Cancer Gene Ther. 2024 Nov 21. doi: 10.1038/s41417-024-00850-z. Online ahead of print. Cancer Gene Ther. 2024. PMID: 39572849
-
The pivotal role of ZNF384: driving the malignant behavior of serous ovarian cancer cells via the LIN28B/UBD axis.Cell Biol Toxicol. 2024 Nov 19;40(1):100. doi: 10.1007/s10565-024-09938-6. Cell Biol Toxicol. 2024. PMID: 39562372 Free PMC article.
-
Integrated proteomics and scRNA-seq analyses of ovarian cancer reveal molecular subtype-associated cell landscapes and immunotherapy targets.Br J Cancer. 2024 Nov 15. doi: 10.1038/s41416-024-02894-2. Online ahead of print. Br J Cancer. 2024. PMID: 39548315
-
DeSide: A unified deep learning approach for cellular deconvolution of tumor microenvironment.Proc Natl Acad Sci U S A. 2024 Nov 12;121(46):e2407096121. doi: 10.1073/pnas.2407096121. Epub 2024 Nov 8. Proc Natl Acad Sci U S A. 2024. PMID: 39514318 Free PMC article.
References
-
- Hu Z et al. The Repertoire of Serous Ovarian Cancer Non-genetic Heterogeneity Revealed by Single-Cell Sequencing of Normal Fallopian Tube Epithelial Cells. Cancer Cell 37, 226–242.e7 (2020). - PubMed
Publication types
MeSH terms
Substances
Grants and funding
LinkOut - more resources
Full Text Sources
Other Literature Sources
Medical